Miguel Lurgi
26 September 2024
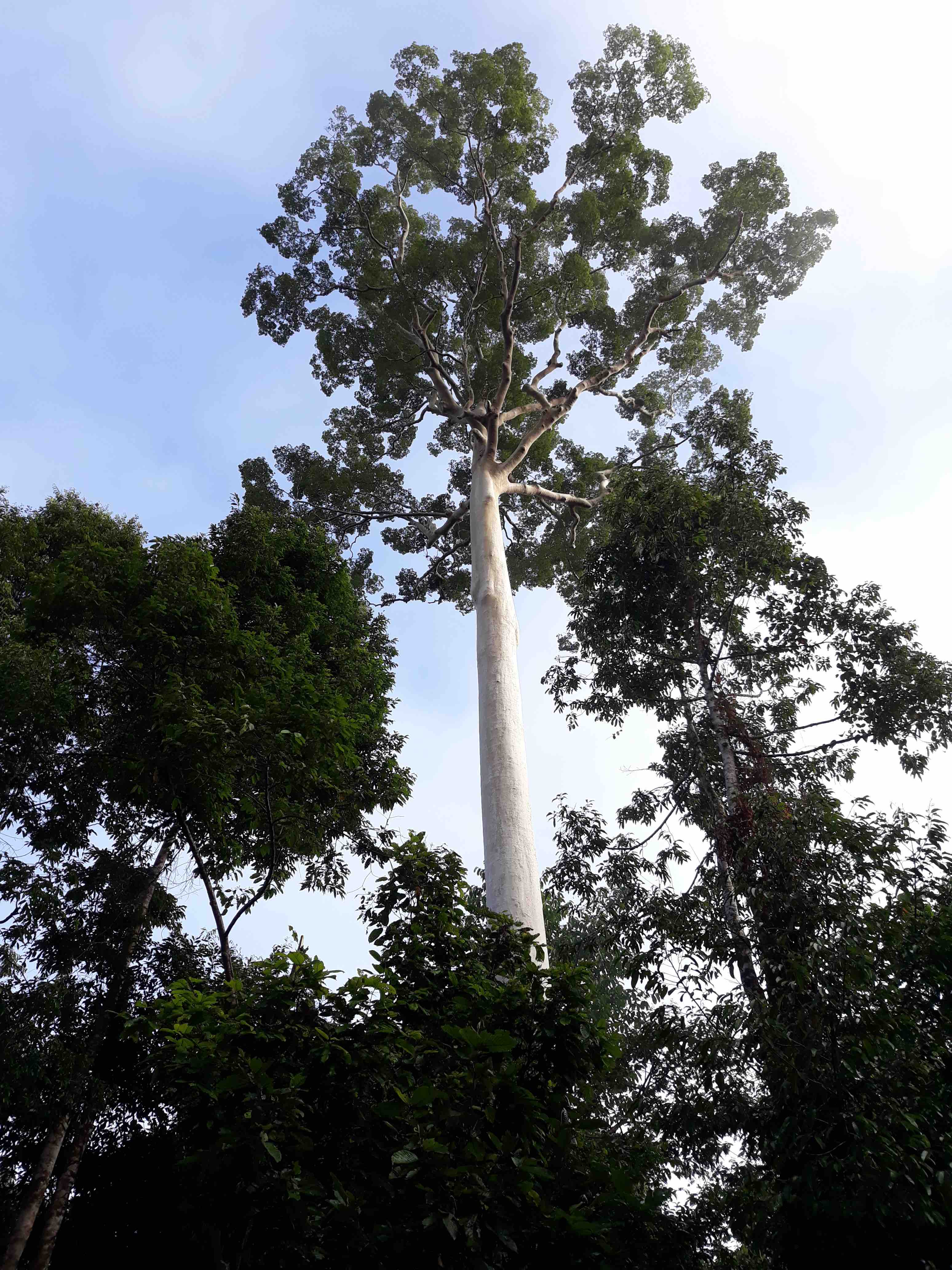
For a couple of weeks from the 15th of September I joined the overseas field course on Tropical Ecology and Conservation in the island of Borneo. Borneo is not only an amazing place for wildlife, sitting right in the tropic and harbouring one of the largest lowland tropical rainforest in the planet. It also sits in the middle of the biogeographical province studied by Wallace and many others since the 1850’s due to their interesting biogeographical patterns, where different branches of the phylogenetic tree collided in what is now known as the Wallace line, separating the Asian and Australian continents faunas.
We spent a few days at the Danum Valley Field Centre, an amazing place for research and teaching that has been welcoming us for a few years now. We developed many interesting lectures and field activities where the students learned about the tropics and the species found there as well as a set of field techniques that helped them with their research projects.
As every year, there are many opportunities for birding, especially when walking around with DeDe, Jonni, and Ahmed, our friends from Danum and whose knowledge of the valley and the species found there is out of this world! They always go above and beyond to show us and teach us everything about the rainforest.
This year, I managed to see around 140 species, with many cool species such as the helmeted, wreathed, oriental pied, and rhinoceros hornbills, the bornean bristlehead, blue-headed and black-crowned pittas or the white-fronted falconet.
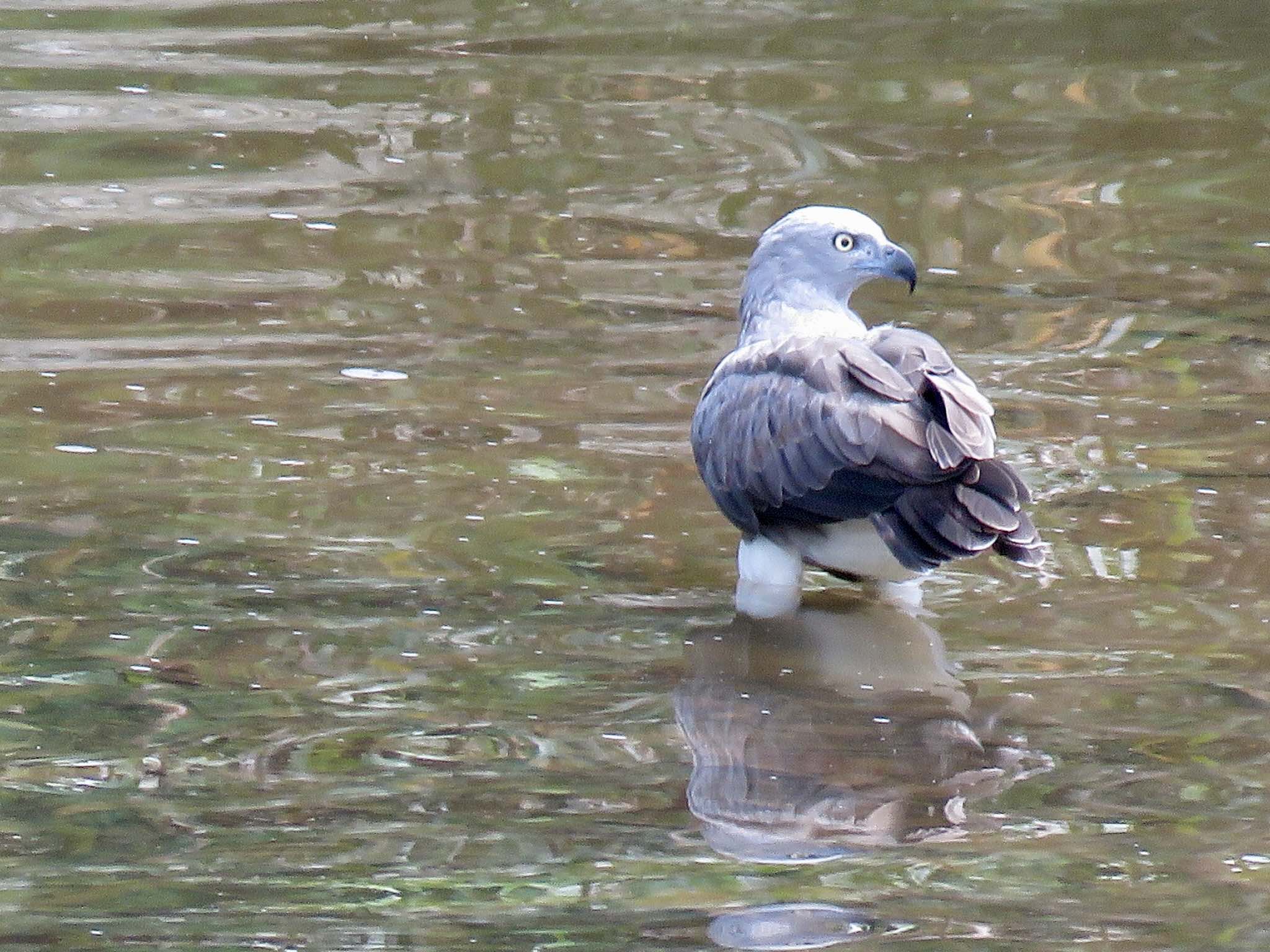
We also managed some amazing views of the grey-headed fish-eagle as well as the Wallace’s hawk-eagle in the nest with a chick!
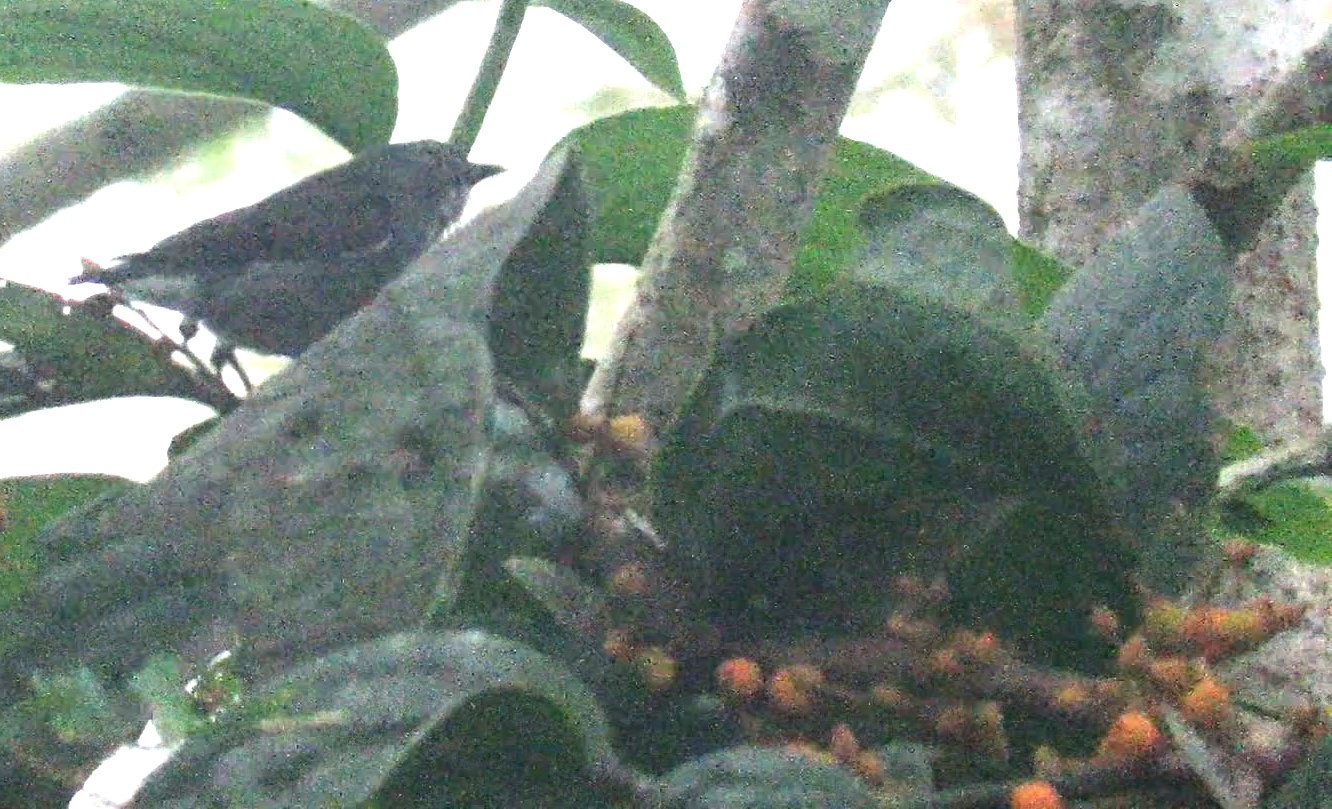
But the highlight of the trip was the elusive spectacled flowerpecker (Dicaeum dayakorum), a species of flowerpecker that had not been seen in Danum Valley since 2009 and never before seen at the field centre where we were based. I first saw this bird just by chance while walking close to the hostel accommodation building. At first I wasn’t sure what species was it, as I had never seen it before. After some research I realised it could be an individual of this rare species, so I convinced a couple of students, Lewis and Joe, to join me in the quest of obtaining photographic evidence of the finding. Surely enough, Lewis managed to capture some images.
I got in touch with some experts in the species, including Professor David Edwards, from Cambridge University, who confirmed the finding. This evidence inspired me to write a small article note to get this observation published. The small article, authored by myself, Lewis and Joe will appear in the December issue of BirdingASIA.
After our time at Danum we also visited the sun bear conservation centre in Sepilok, where I met a lovely Catalan couple who had been travelling by car around Malaysia for a few months, and I had the chance to practice my Catalan with them! The visit to the bear sanctuary, adjoining with the orang-utan conservation centre, was an interesting experience for the students to see how local conservation projects are set up and what they can achieve.
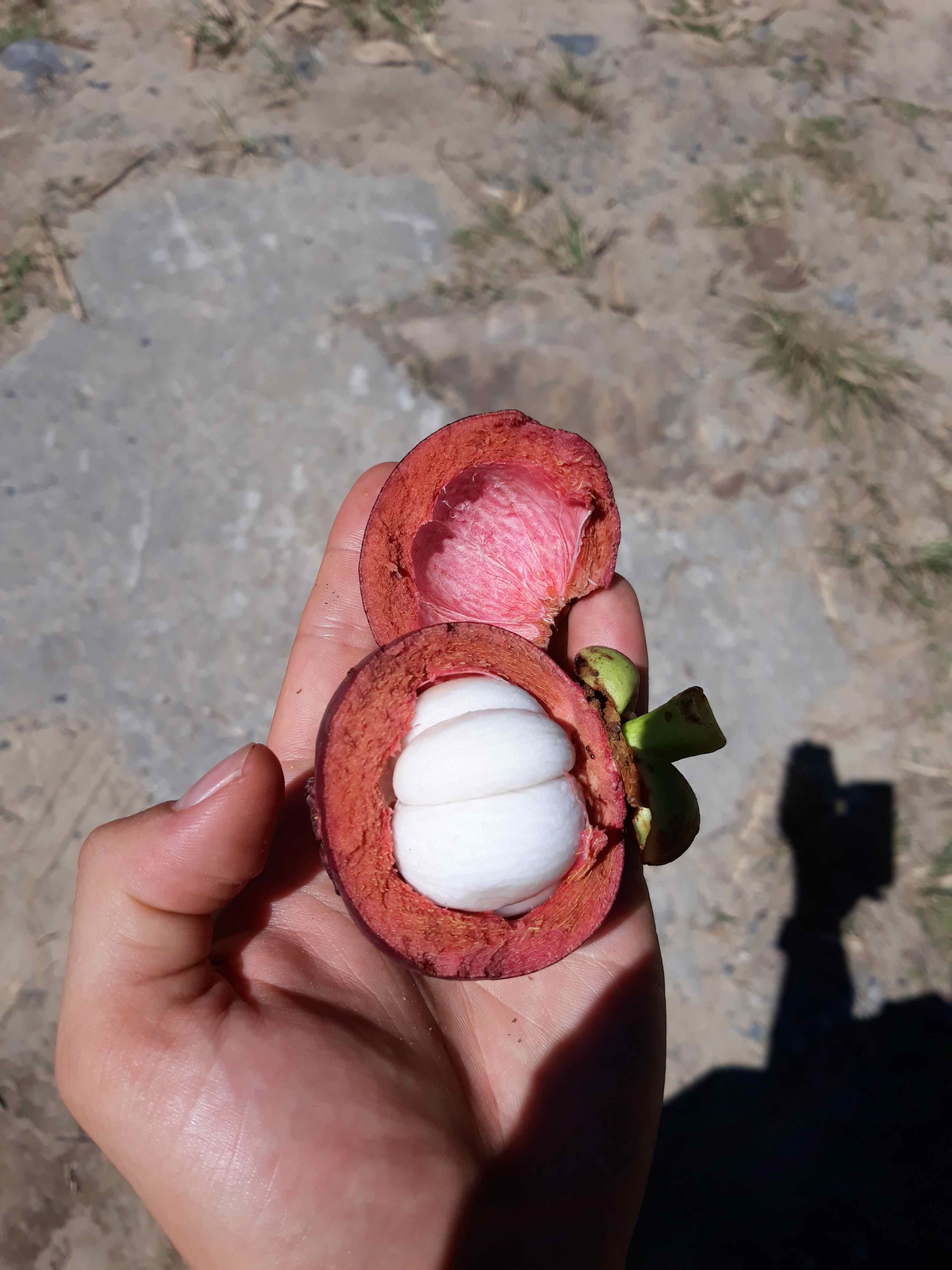
After Sepilok we moved on to Poring to find one of the jewels of the Bornean flora, the Rafflesia flower, a parasitic flower that is pollinated by flies, and as such produces an aroma similar to the smell of rotten meat to attract its pollinators!
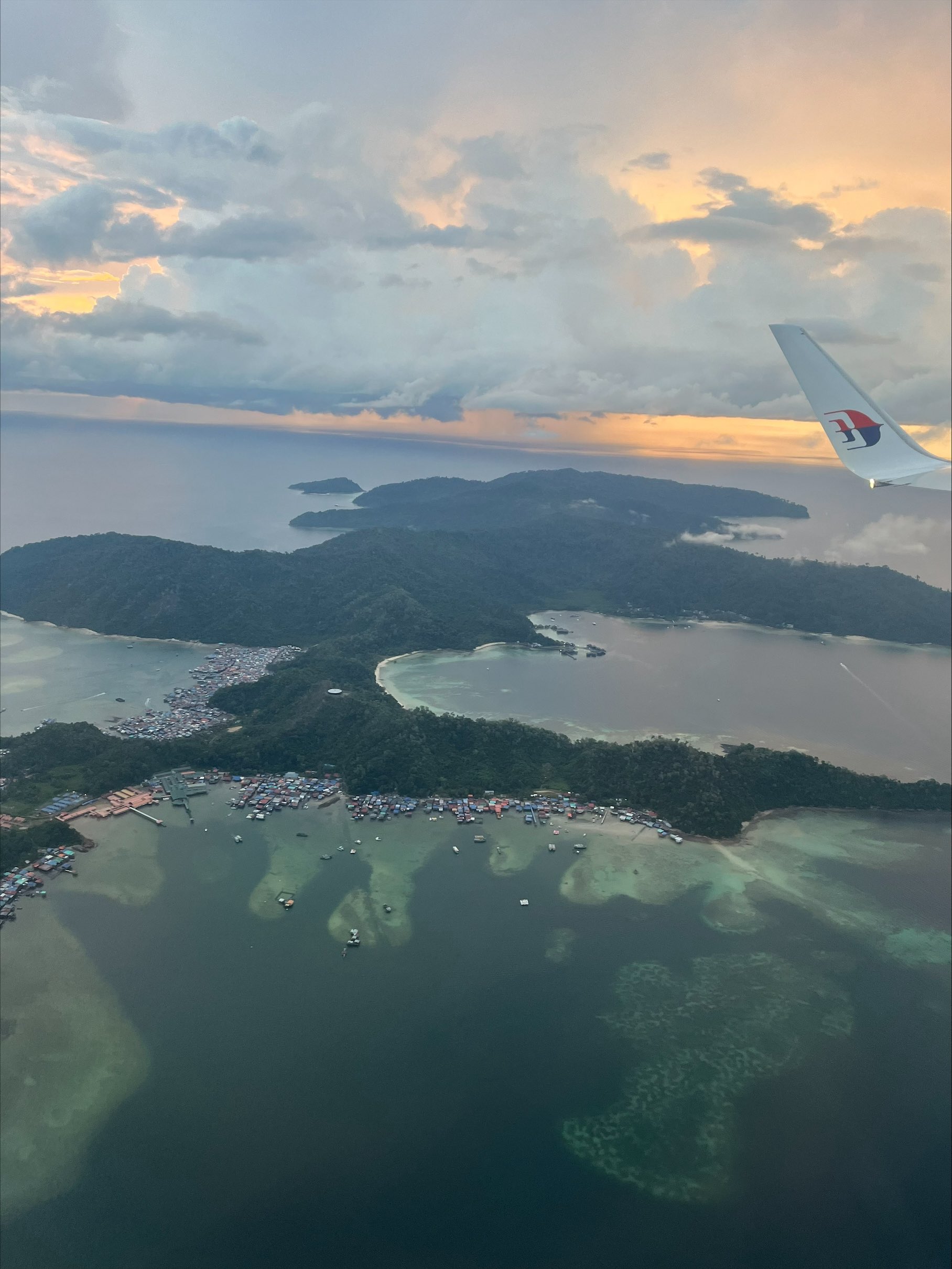
At Viviane’s Rafflesia garden we also had the chance to see some local plants such as rambutan and mangosteen, from which trees we harvested ourselves some fruits and ate them there by the trees!
Our last stop before heading back home was the Mount Kinabalu. We visited the botanical garden of Mount Kinabalu, where we encountered many interesting plants, including the smallest orchid in the world! as well as some interesting birds such as the Bornean tree-pie, the Bornean whistler, and the Temminck’s sunbird.
We then flew off from Kota Kinabalu, saying good by to the happy times we had in Borneo!
Thanks to Jonni, Ahmed, DeDe, Sesan, Louis, and all the other RAs at Danum Valley for a great couple of weeks!
Photo credits: Lewis Ferguson - All pictures of birds and the view of the island from the airplane
Miguel Lurgi
20 August 2024
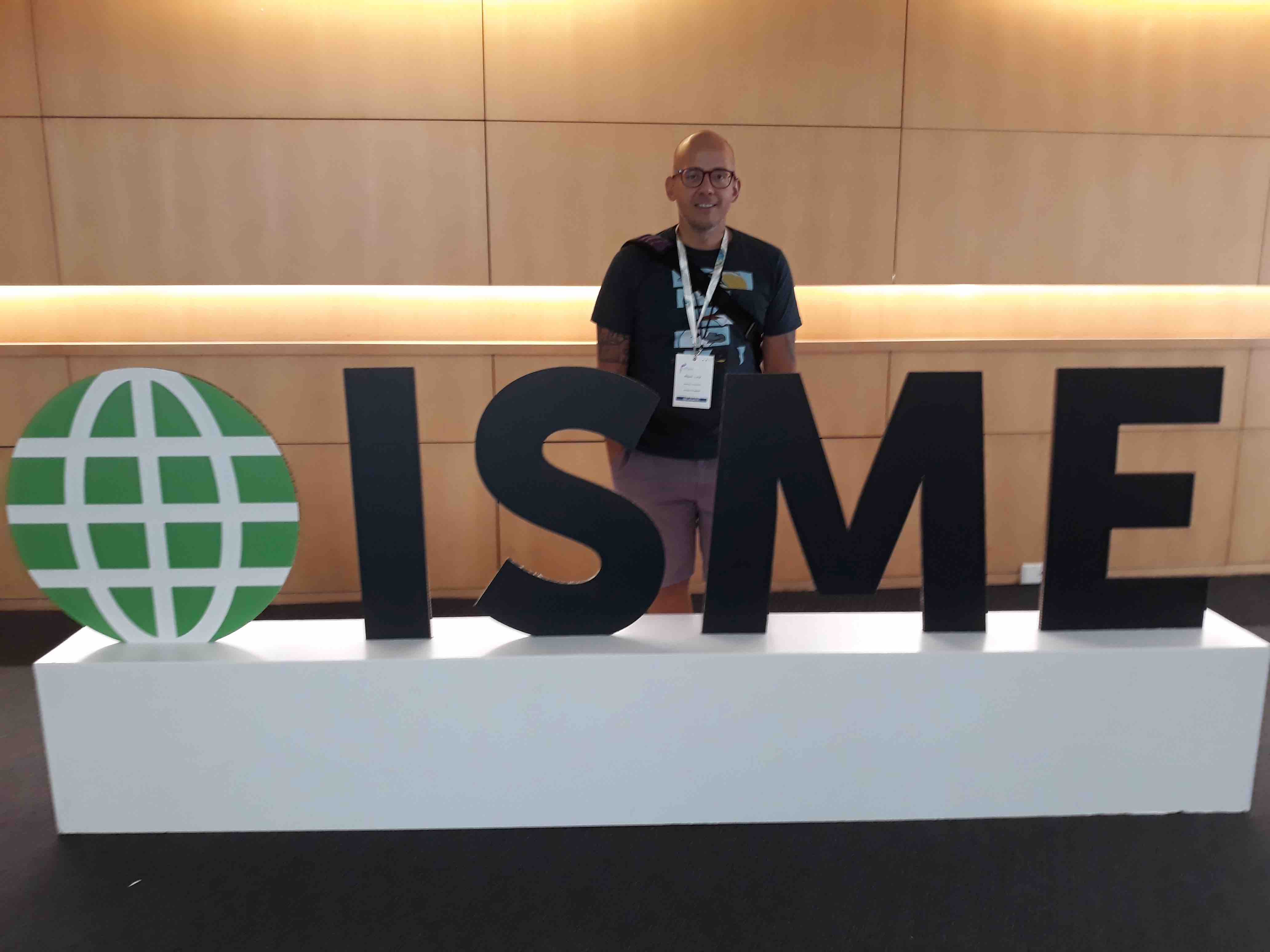
This week we are at the 19th Symposium of the International Society for Microbial Ecology - ISME in Cape Town, South Africa! Thanks to the support of the Leverhulme Trust, through my current Research Project Grant, The origin of complex symbioses were were able to share our current findings with microbial ecologists from around the world!
I presented our research on the role of mutualisms on the eco-evolutionary assembly of complex microbial communities.
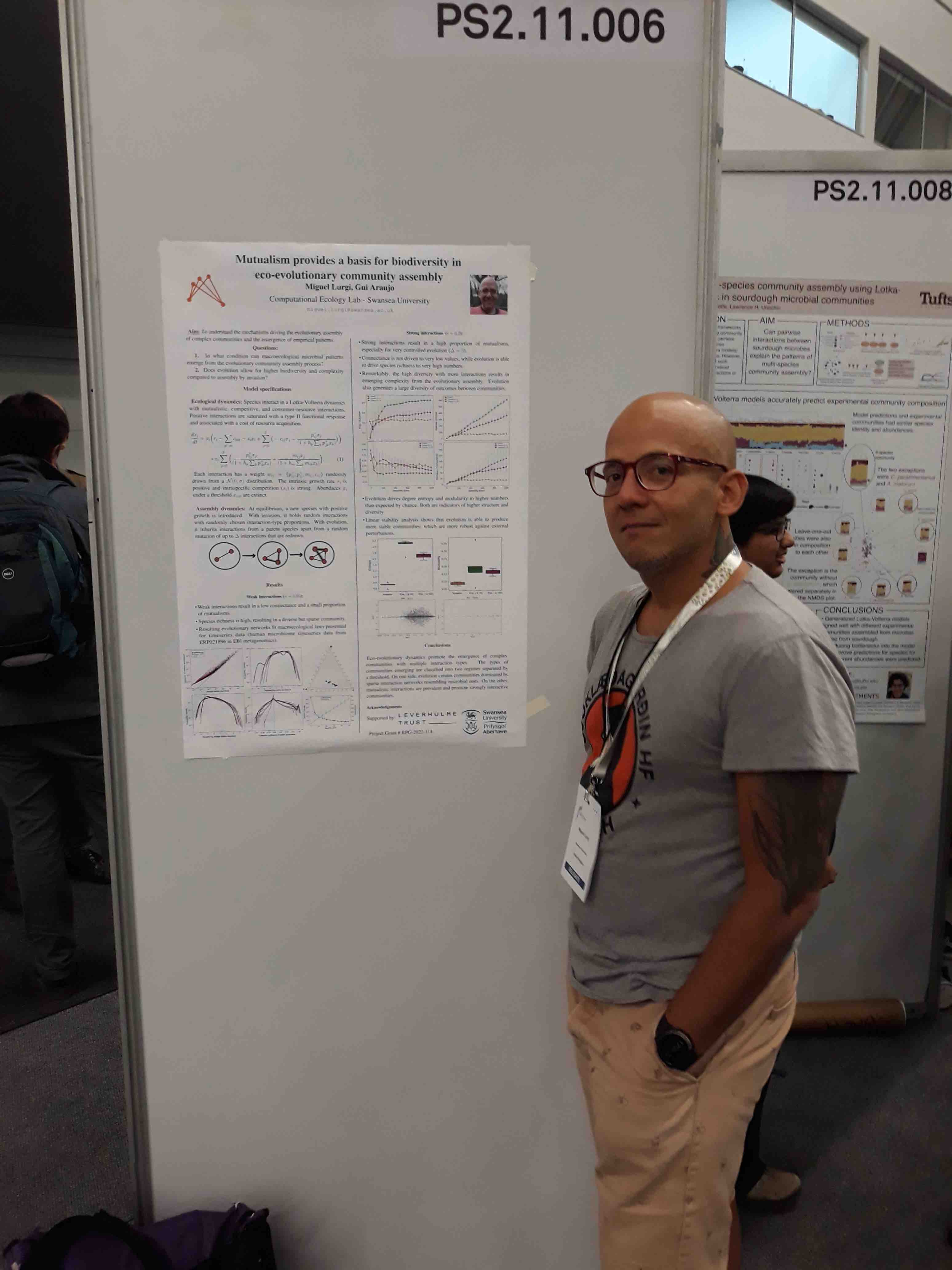
Abstract:
Understanding the diversity and structure of microbial communities is a central focus of microbial ecology. Recent research has unveiled their organisational patterns, highlighting the sheer diversity found within and across them. Identifying the mechanisms behind these patterns is fundamental to develop an understanding of microbial community assembly. Experimental and empirical evidence suggests that both ecological and evolutionary mechanisms influence microbial community assembly. Especially considering the coupling of timescales between ecological and evolutionary processes in that is unique to microbes. However, a comprehensive understanding of how ecology and evolution come together to create complex microbial assemblages is still in its infancy.
In this work, we aim at filling this gap by developing an eco-evolutionary model of microbial interaction networks. We account for the interplay between ecology and evolution by considering interaction-driven population dynamics and evolution through speciation and inheritance of interactions. The model is capable of generating complex communities with many species and interactions typical of microbial assemblages. Moreover, by modulating the ratio of interaction types we can obtain a variety of community structures, thus generating a diversity of community types. We found that mutualistic interactions, by making communities more stable, allow communities to accommodate a larger number of interactions overall. We compare our findings to a purely ecological model and show that ecological assembly is not enough to generate complexity patterns such as those attained by evolution.
Our results are relevant for generating predictions on the effects of perturbations on natural microbiome and developing strategies for engineering synthetic microbial communities.
This diversity is manifested at several scales. Not only are microbial communities highly diverse, but they also display different structures that varies across environments, biomes, or host types.
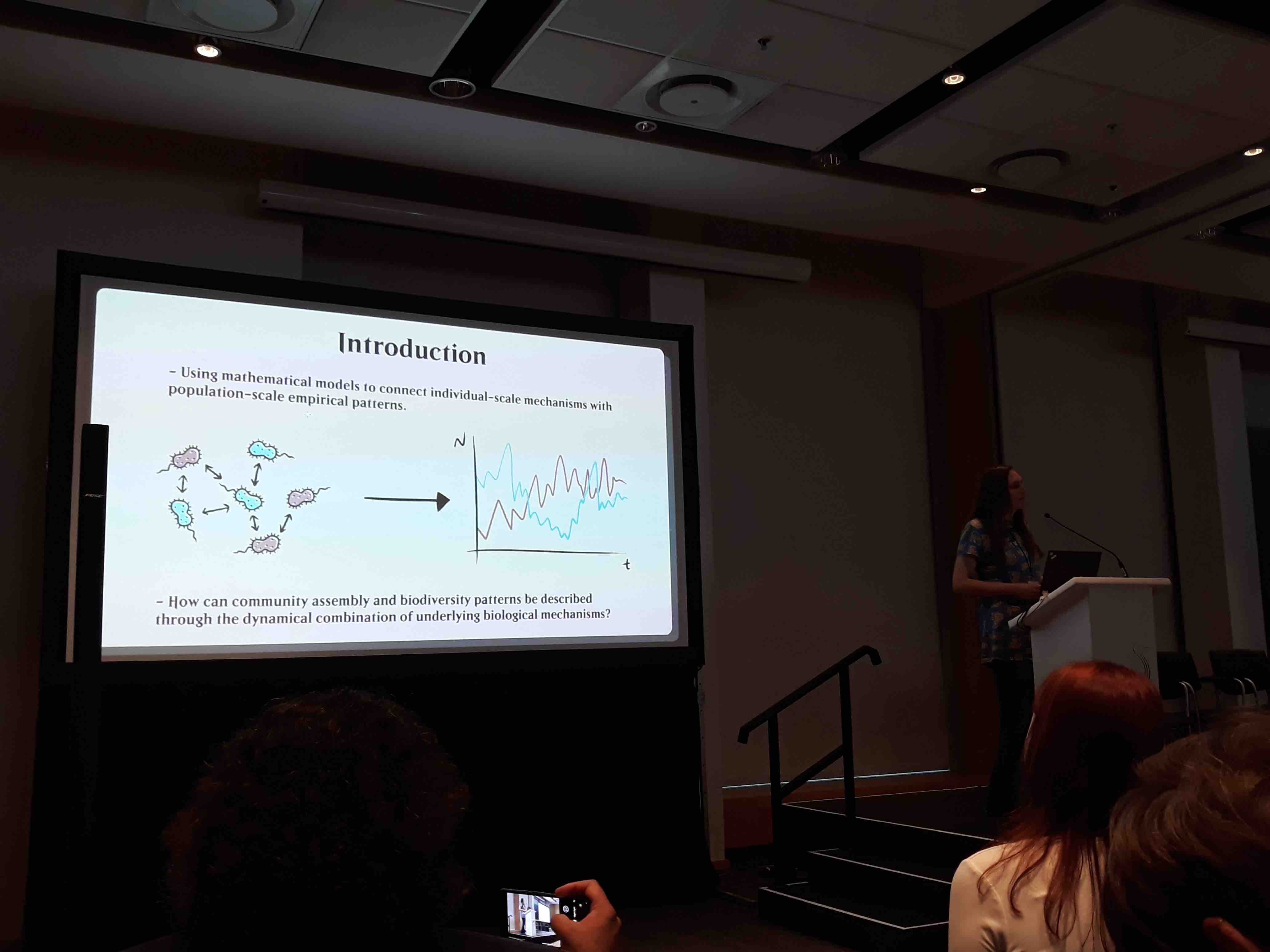
Gui expanded on this work and presented our current joint work on a spatial meta-community framework for complex microbiomes.
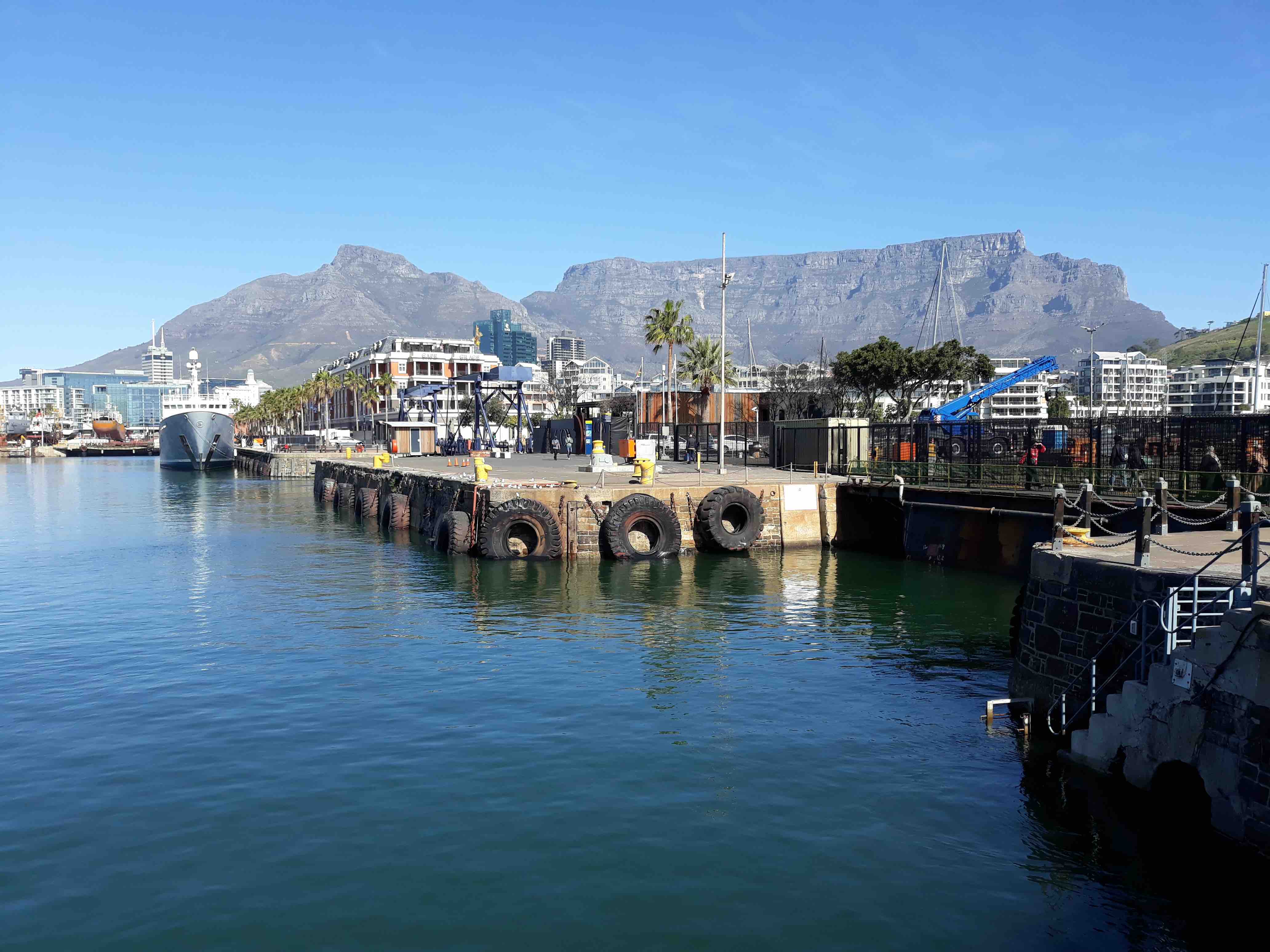
The opportunity to see some exciting new research in microbial ecology was fascinating, with many interesting talks and poster presentations. Plus the chance to catch up with old friends from Australia and newly made friendships!
Thanks to Heidi, Nicole, Bettina, Paul and Mike for many nice times and interesting science discussions! It was great to see many of you again!
Miguel Lurgi
10 July 2024
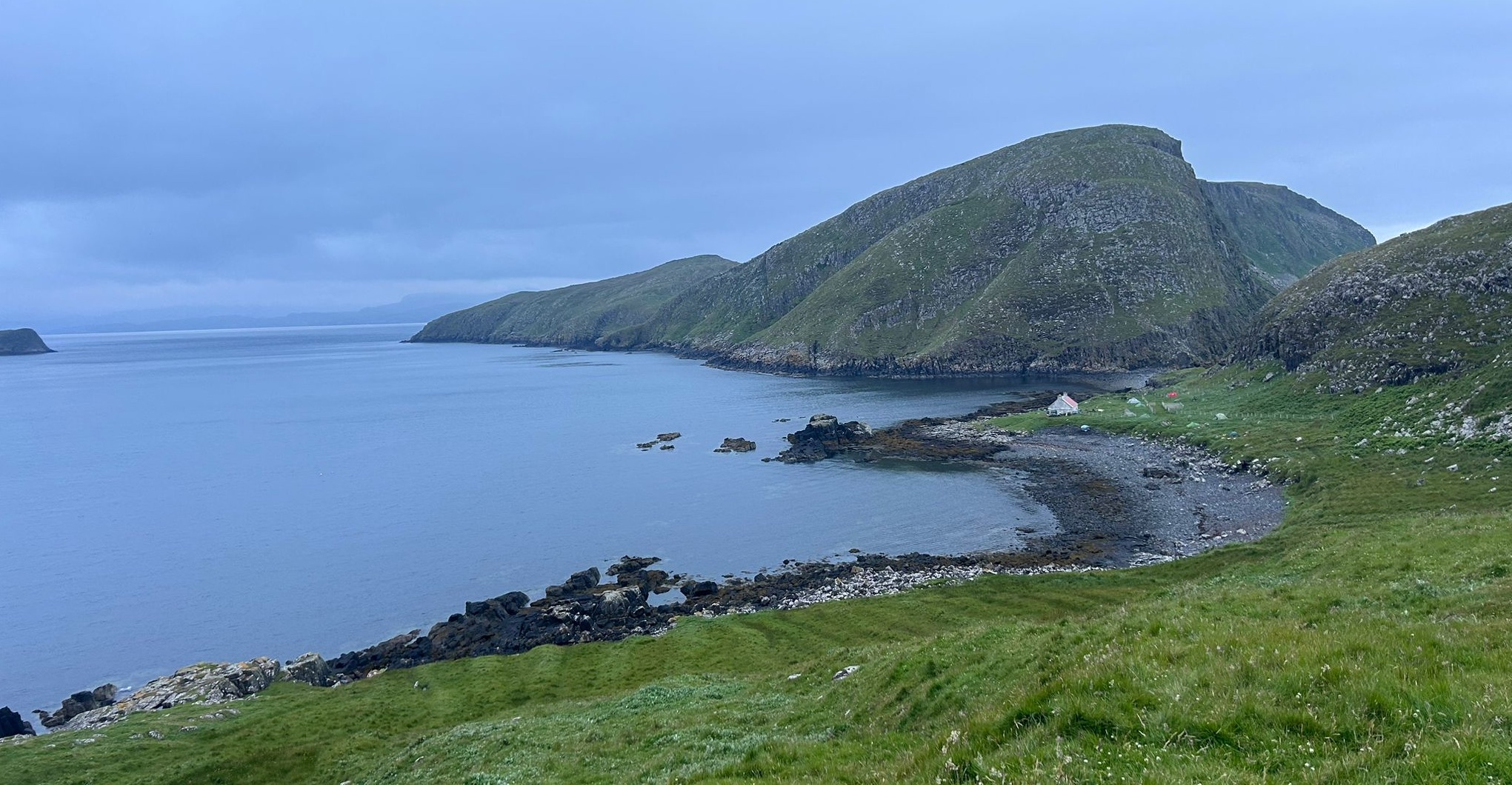
This summer, thanks to the support of the British Trust of Ornithology (BTO) through their Seabird Ringing Grants, and the folks at the Shiants Seabird Research Group, I had the once in a lifetime opportunity to spend one magical week on the Shiant Isles searching for birds.
The research that the Shiants Seabird Research Group does on the isles contributes to national and international efforts to better understand the ecology and population dynamics of these amazing creatures. It was an honour to have the opportunity to be part of this project and contribute to their ringing activities. I had the chance to work with an amazing group of people on one of the nicest places on Earth!
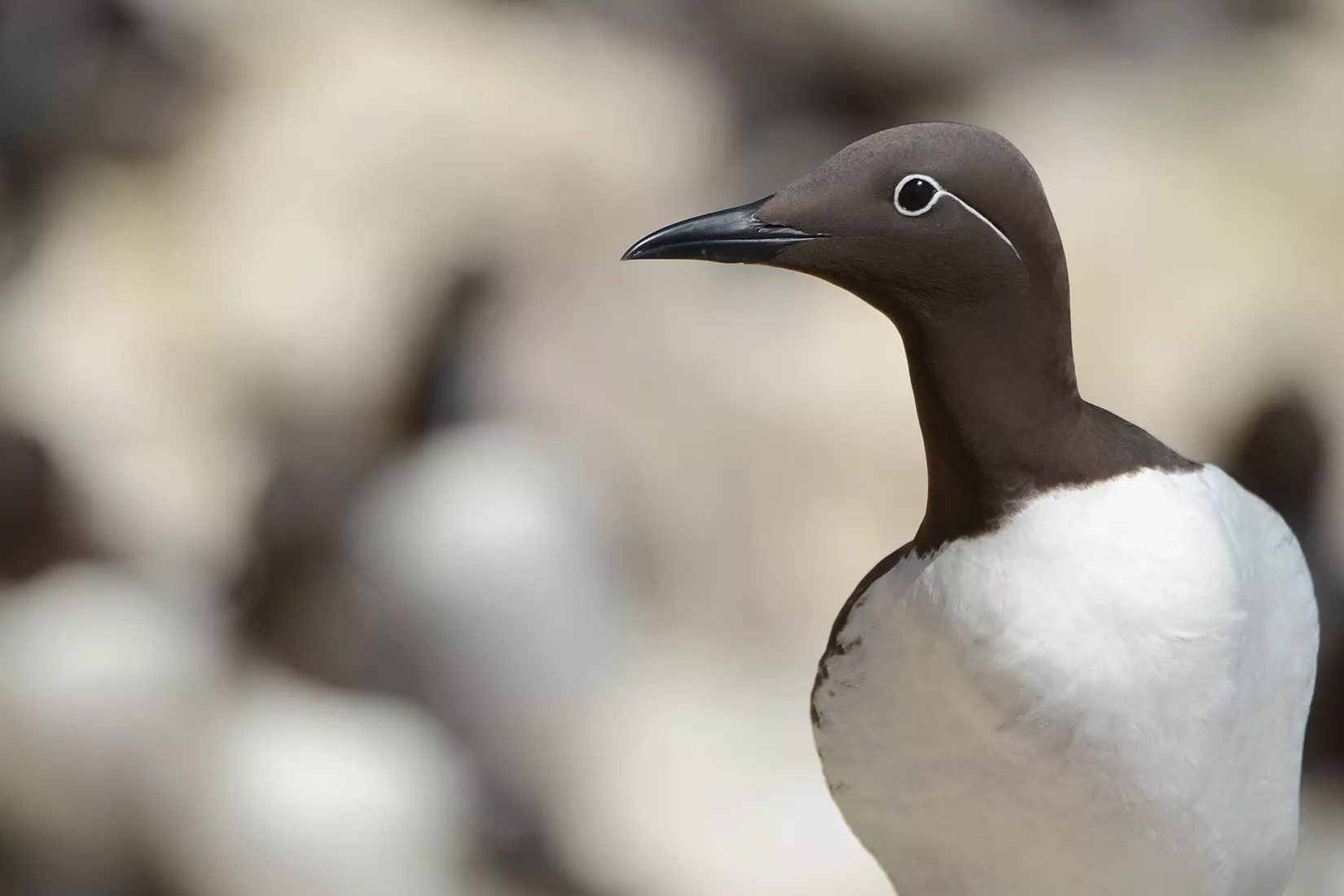
I will be forever thankful to the BTO for their support and the Shiants Searbird Research Group for welcoming me into the group during the week and making me feel like one of their own. I had a great time sleeping in a tent for the whole week, getting water from the well, helping out to move the dinghy about, and just in general being in an amazing and remote location “off the grid”!
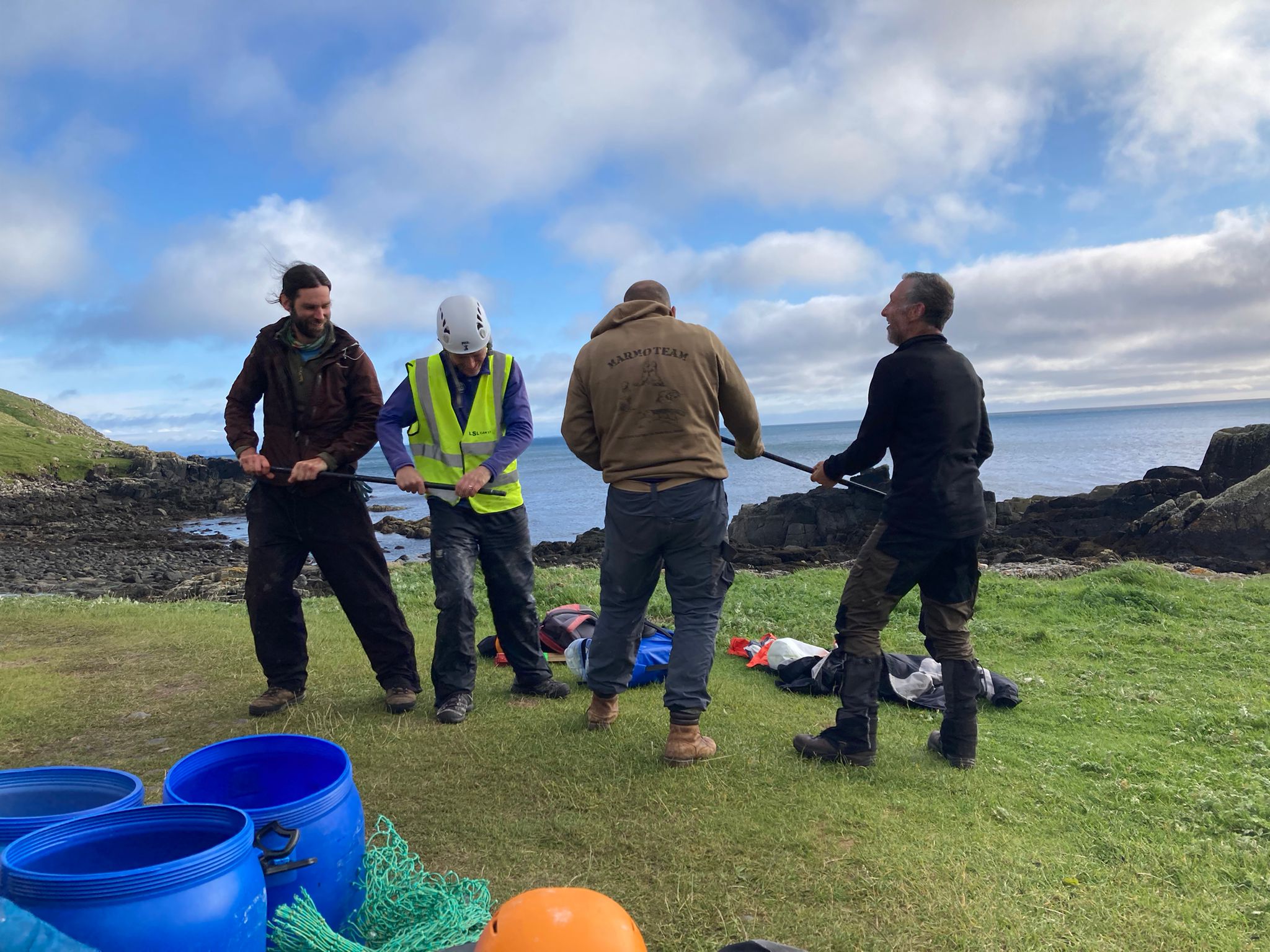
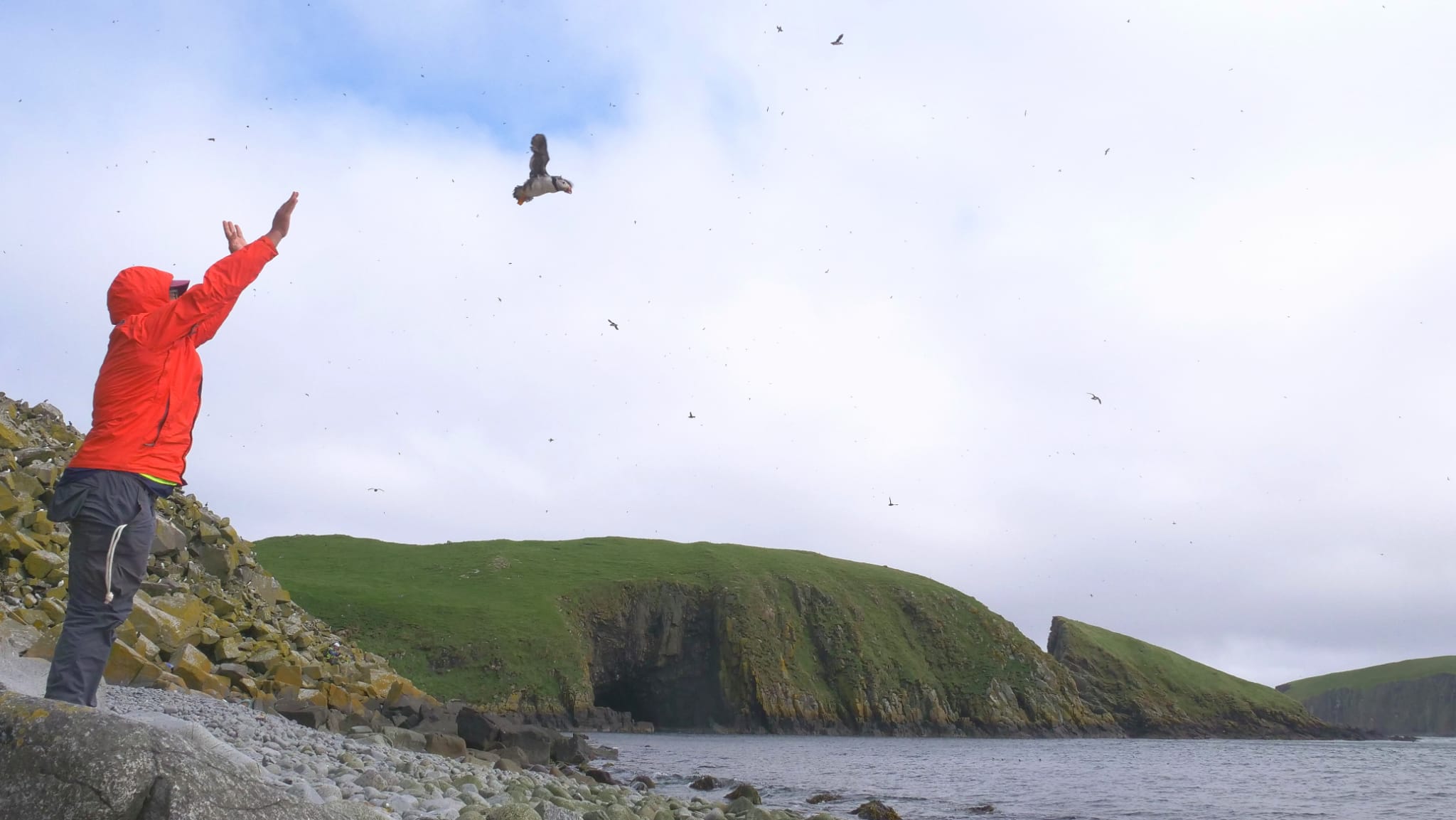
And of course, the wildlife. Species seen included white-tailed eagle (Haliaeetus albicilla), razorbill (Alca torda), common guillemot (Uria aalge), arctic tern (Sterna paradisaea), great and arctic skua (Stercorarius skua and Stercorarius parasiticus), european storm petrel (Hydrobates pelagicus), atlantic puffin (Fratercula arctica), european shag (Phalacrocorax aristotelis) among other nice birds!
I had the chance to ring species such as razorbills, guillemots, skuas, shags, artic terns, and puffins! All in all, amazing!
Thanks to Jim, Noelia, Rhys, Rhys2, Ian, Rosie, Dean, Sophie, Liz, Andy and Carole for the great times!
Miguel Lurgi
10 June 2024
This week we are at the Mediterranean Institute for Advanced Studies (IMEDEA) of the CSIC in Mallorca! Thanks to the Maria de Maetzu Visiting Scholar Programme, I was able to spend some time here and discuss some exciting research with Research Professor Anna Traveset on the ecology and biogeography of pollination and seed-dispersal networks.
Lucie joined us for a couple of days and she also had the change to discuss with Anna and members of her group the exciting research they are doing as well as Lucie’s own work on the modelling of the spread of disturbance on spatially extended food webs.
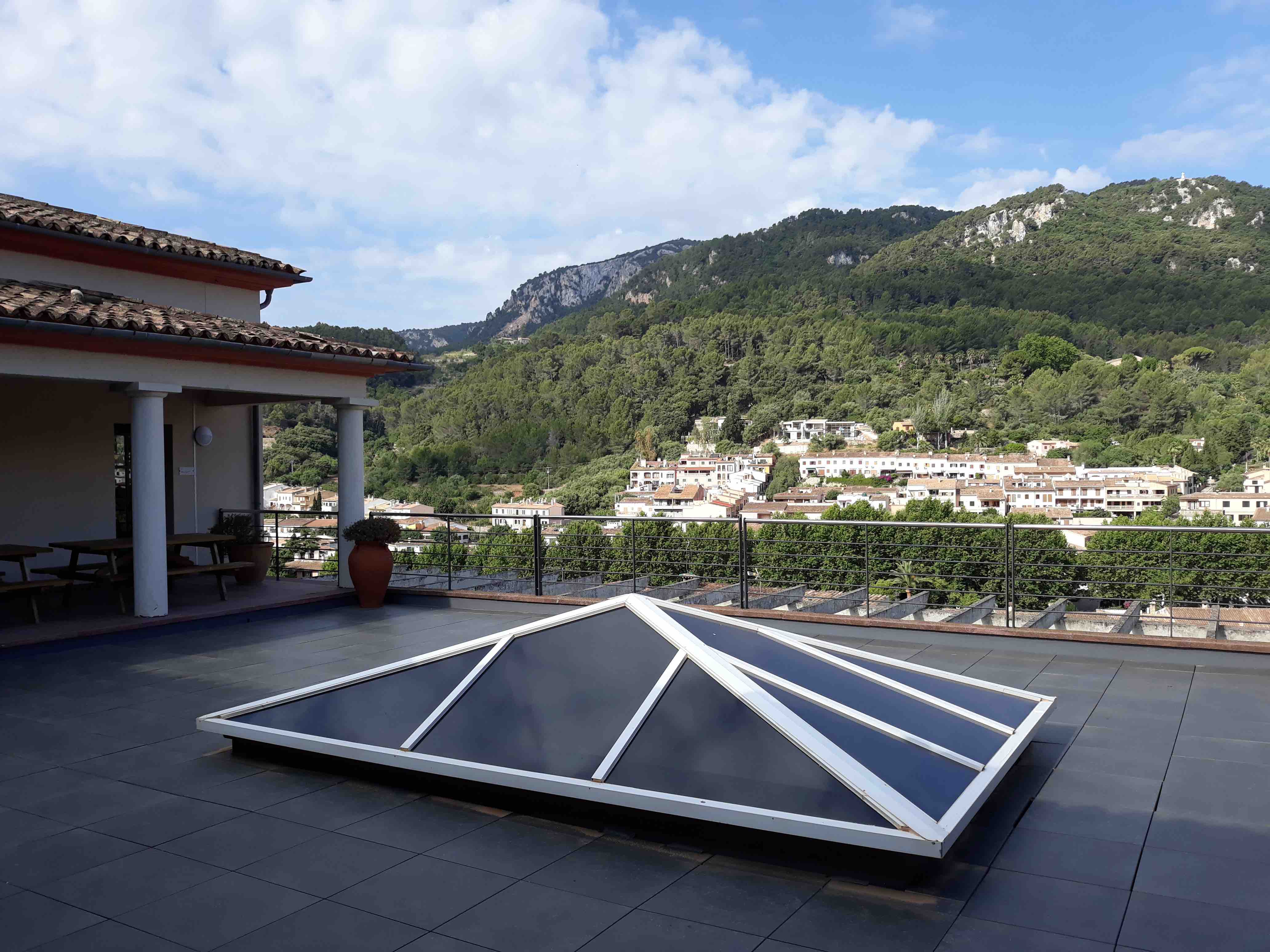
Anna kindly organised for me to give a seminar on Understanding the network structure of ecosystems to guide conservation action where I shared our current research on microbial communities and the understanding of the effects of protected areas on food web structure.
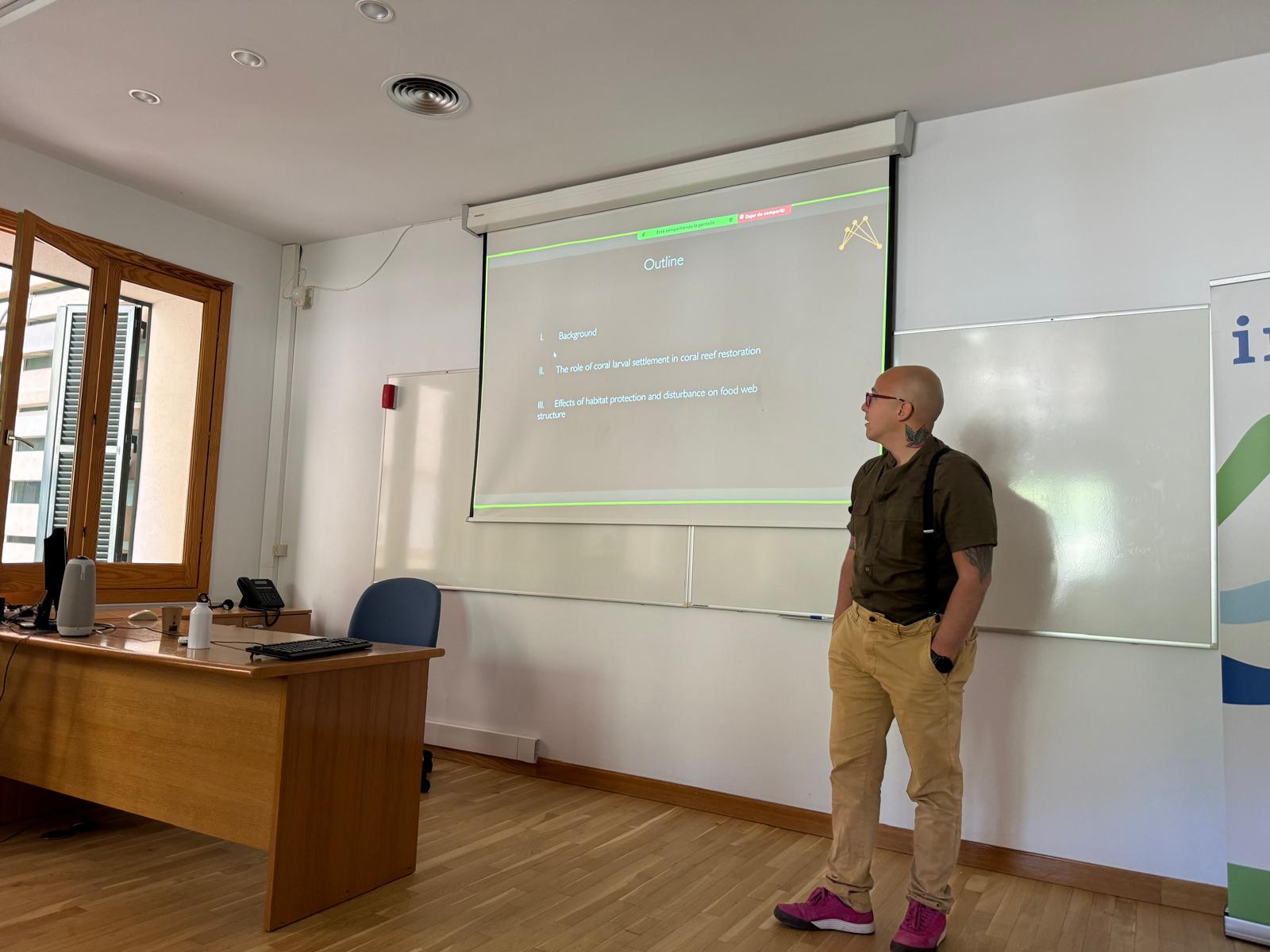
We not only enjoyed the research but also the amazing food and some afternoon hikes around the Esporles surroundings!
Thanks to Anna, Isa, Alba, Max, Andrea, Manolo, Giacomo and many people @ IMEDEA for the great times!
Miguel Lurgi
27 May 2024
This week our time in Australia comes to an end with a visit to my long-term research collaborator and friend Prof Nicole Webster. Nicole is a reserach scientist a the Institute for Marine and Antarctic Studies of the University of Tasmania.
From a microbial ecology and evolutionary perspective of the sponge-associated microbiome, she is helping us develop the theoretical framework for the assembly of complex symbioses that we are currently building as part of my project The origin of complex symbioses funded by the Leverhulme Trust.
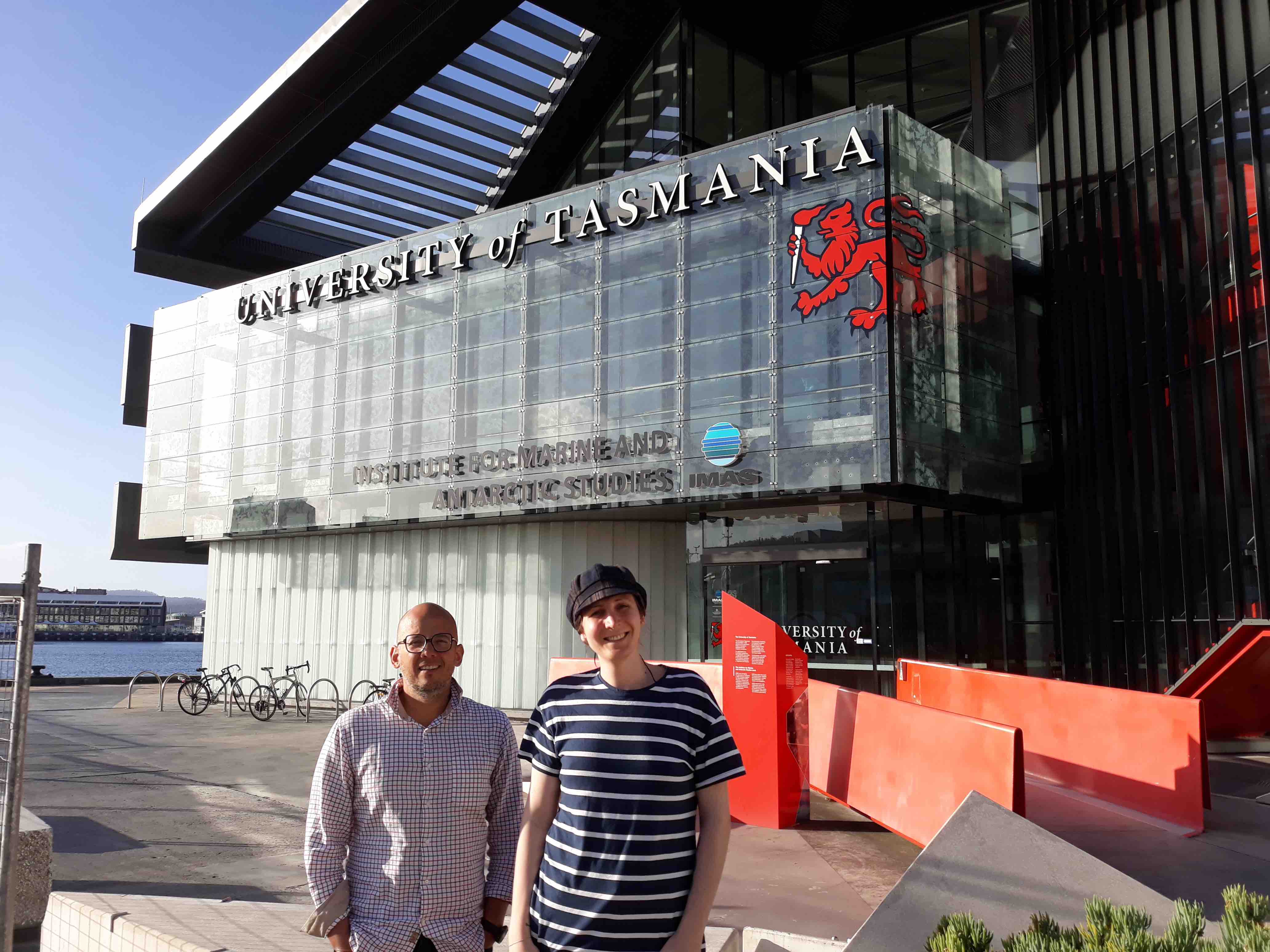
We have had very interesting discussions with Nicole about the ecology and evolution of these complex symbiotic associations and how we can incorporate different different evolutionary and ecological mechanisms into a mathematical framework to better understand their emergence.
Nicole and Sophie also kindly organised a seminar to showcase the research we carry on in the lab. The seminar was entitled: Network perspectives on community assembly and disassembly and you can read the abstract below.
Abstract
Quantifying general patterns of community structure, and the mechanisms behind them, is key to understand the persistence and collapse of complex ecological communities. To achieve this, we should develop holistic approaches that not only consider species composition but also ecological interactions between them. We tackle this challenge by unveiling empirical patterns of species interactions networks alongside theoretical approaches that enable a better understanding of their assembly and disassembly.
In this talk, I will present empirical and theoretical examples of this approach applied to microbial marine and terrestrial vertebrate systems. On the microbial side, we explore the relation between biofilm microbial network structure and the successful settlement of coral larvae. We complement this with a mechanistic theoretical approach to microbial community dynamics in the host-associated microbiome to investigate the emergence of host types in marine sponges. Focusing on terrestrial avian communities, we assess the beneficial effects of protected areas on food web structure and relate these changes to considerations of biomass flow across the ecosystem. Lastly, I will present a theoretical approach to predicting Network-Area relationships. This contributes to existing knowledge on the spatial scaling of biodiversity.
Thanks Nicole and Sophie for the warm welcome and the great time @ IMAS!